Last week, we discussed the five pillars of successful analytics initiatives. The week before, we made the case for Analytics as a business process. Today, we will talk about the third and last foundational element of successful analytics workflows: communication.
Speaking different languages…sometimes literally
Everyone talks or writes about the need for good communication between business leaders and analytics team, but what does it mean exactly? And what is really the issue?
Well, fundamentally, the issue is that the business side and the analytics side speak different languages. In other words, the business side communicates via what computer scientists call “natural language”, aka plain English. Meanwhile, the analytics side speaks “math & stats”, the language of science, made up of equations and ancient Greek characters.
In real life, a typically conversation between a business leader and an analyst would look like this:
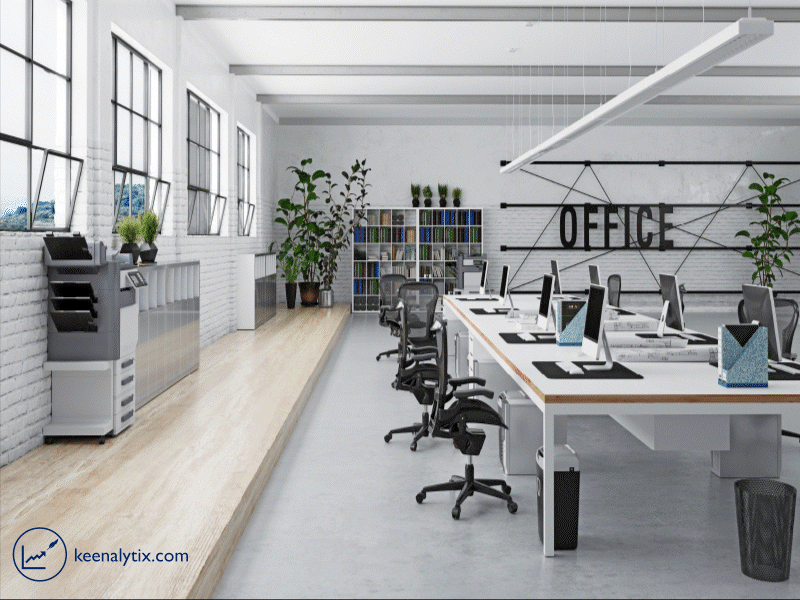
…which would inevitably lead to this:
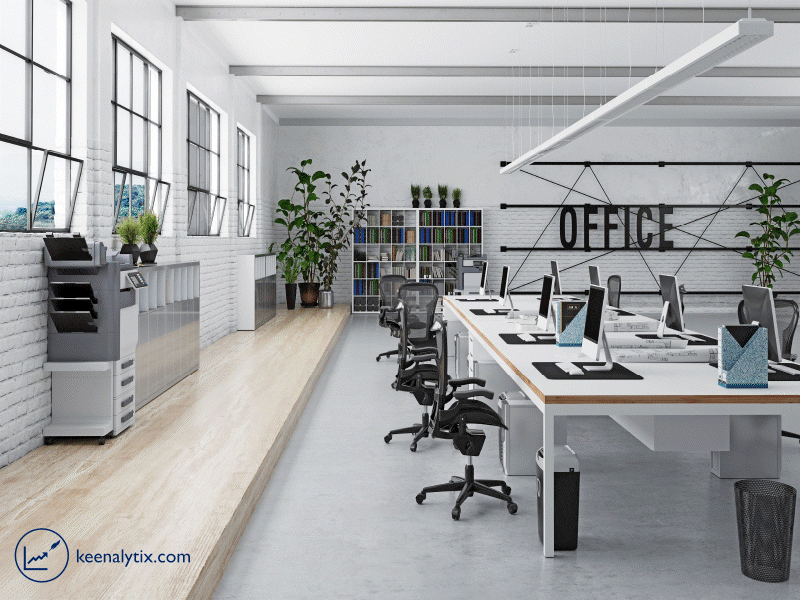
To be clear, the disconnect does not come from the use of different languages per se. There are about 4500 languages and dialects spoken on the planet. It has never prevented human beings from communicating with each other and getting things done.
The main challenge here is that natural languages are full of ambiguities, something the scientific language does not allow. Among others, the same word (take, for instance, the word “leverage” from the first illustration) can have multiple meanings, depending on the context. In science, once you have defined what “alpha” means (for a given problem), its definition does not change.
Inevitably, when the two sides try talking to each other directly without any filter, misunderstanding ensues, preventing the business from using its analytics to make data-driven decisions.
The tried-and-tested solution: lexicon and interpreters
To solve this problem, human beings have been following the same approach for thousands of years:
- Develop a lexicon
- Train interpreters
Developing a business/analytics lexicon
A lexicon addresses the need for written references, in both languages. In other words, the lexicon is there to try and remove as much ambiguity as possible and come up with some sort of universal common understanding.
In the case of a business/analytics lexicon, it means the following:
- From business to analytics: the lexicon is there to help the analytics side understand what the business side needs. This is easier than it sounds, primarily because the problems a business are trying to solve (by using analytics) are, to some extent, always the same.
- From analytics to business: likewise, the lexicon can help the analytics effectively communicate its results (and their underlying models) back to the business, especially for the models used to solve repetitive business problems.
The last mile of business/analytics communication: interpreters
The lexicon will likely take you 80-90% of the way there, especially for everything unambiguous enough to be written down by either side. However, the remaining 10-20% is harder to address because it touches upon where most of the ambiguity lies: in oral communication. Enter the interpreters.
In linguistics, interpreters are the trained professionals dealing with oral communication, very often in real-time (as opposed to translators, who deal with written materials). Their job goes above and beyond simply knowing two (or more) languages. They are also experts at understanding and deciphering context, body language and other cues.
In a business/analytics setting, interpreters have to be fluent in both languages. Most importantly, they are there to understand what each side needs to be successful and convey those needs to the other side in terms they can understand. Going back to our illustration, the situation would now look like this:
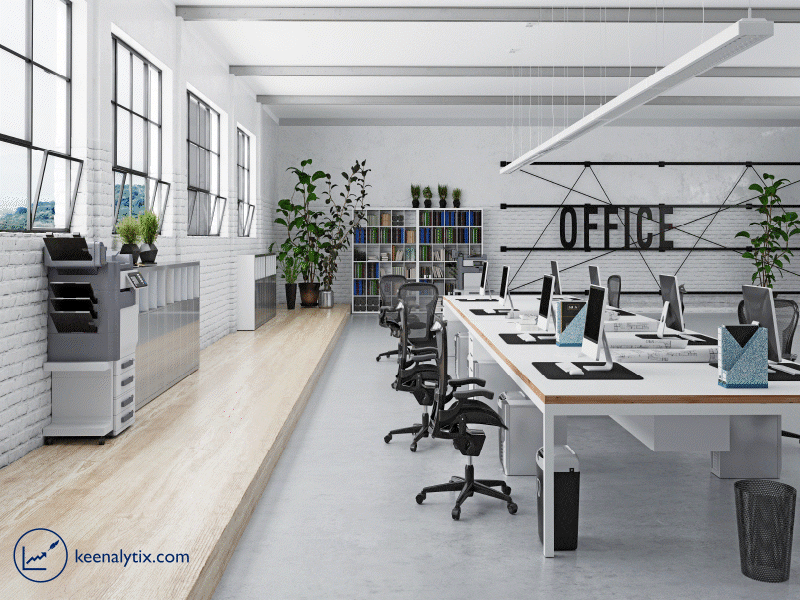
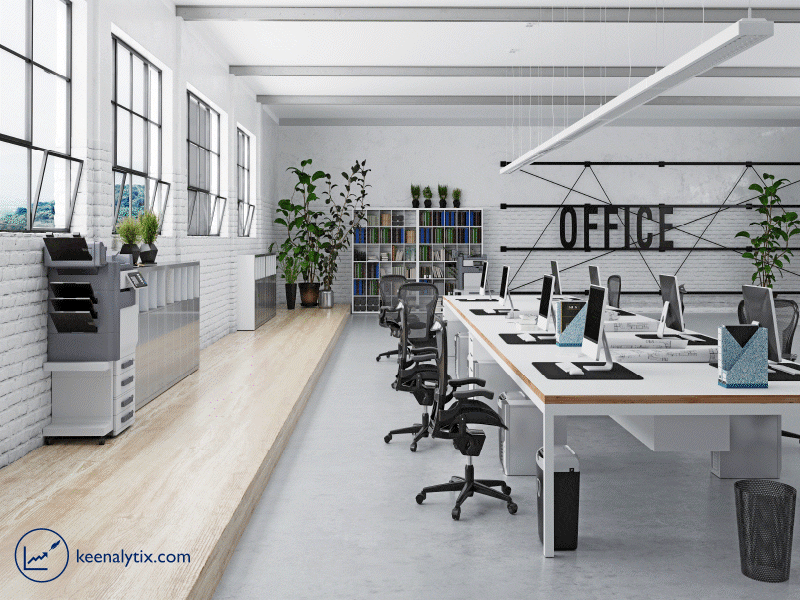
In the initial phase of the project, the interpreter removed the ambiguity from the business leaders’ statement to set the analyst after the right problem to solve. Once the analyst is done, the interpreter is there to help communicate the results so the business leader can make an informed decision.
Business first!
There is, however, a significant difference between dealing with two natural languages and addressing the business/analytics communication challenges. In the business/analytics context, the latter is at the service of the former. In other words, the needs of the business side always take priority. The communications efforts always have to keep in mind that the end game is a business problem to solve, not just an analytics model to build.
This addresses a question we get asked often: who should make the first step? Who should make the effort to learn the other side’s language? We do believe that the effort should be shared. Businesses would benefit greatly if their leaders would speak just enough analytics to at least set their teams on the right track. However, most of the effort should fall on the analytics side, precisely because it is at the service of the business side and not the other way around.
Putting it all together
This concludes our trilogy of foundational elements of successful analytics. To sum it up:
- Analytics is successful when it is executed as a process, not as a succession of ad hoc projects.
- Analytics relies on much more than just data and software. It is also about culture, processes, and skills. Together, they are the five pillars of successful analytics.
- Analytics needs good communication with (and to) the business side, which requires the development of a lexicon and the training of analytics/business interpreters.
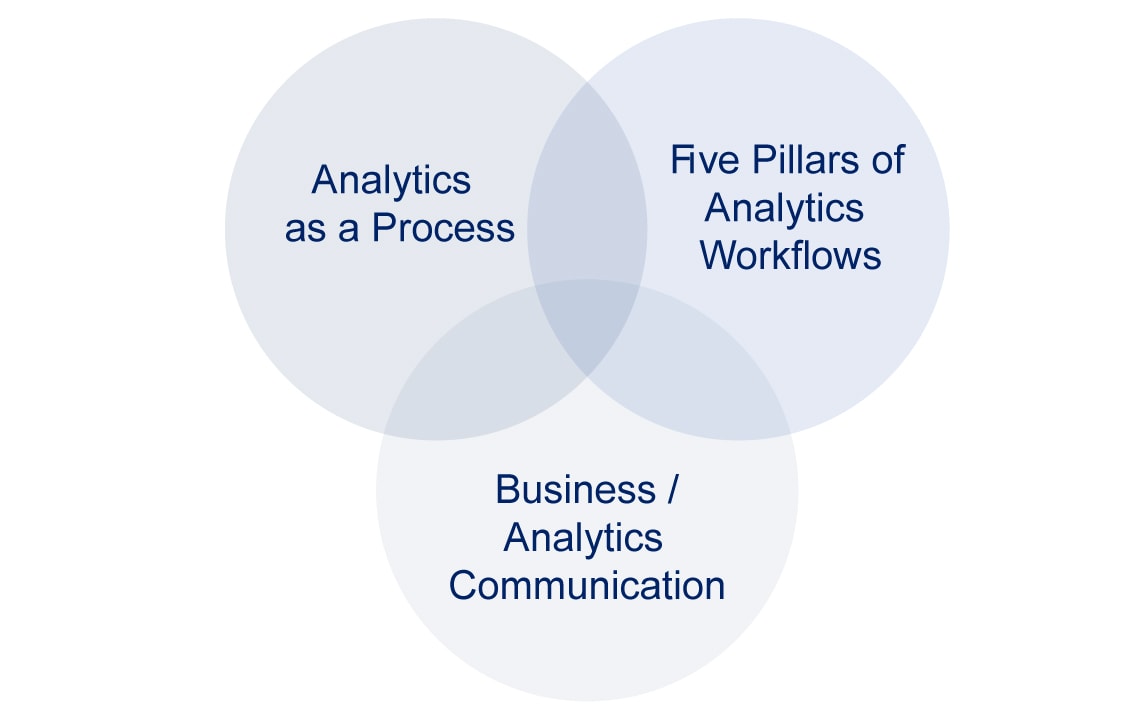