Most analytics projects fail
According to most estimates, more than 80% of analytics initiatives end up being abandoned or fail to deliver the expected value. If you are a business leader (or a data scientist), chances are you have experienced it yourself.
Our view is that most projects fail because Analytics is not treated as a business process, unlike Sales, Marketing or Operations. As a result, and most importantly, analytics projects are primarily “science” initiatives, with a very limited role for the business side.
This gap is apparent in the existing project frameworks used in the industry, typically based on CRISP-DM and/or the INFORMS’ CAP curriculum. With slight differences, existing frameworks see analytics projects like this:
A typical Analytics project:

To be clear, the overall logic of these frameworks is perfectly sound. You obviously do need to start with a problem, tackle it with your knowledge, and end with an acceptable solution. However, the issue lies in the imbalance between these various steps, and the role played by the stakeholders involved.
In other words, under the current frameworks, the business is here to ask the question at the beginning, stay out of the way while the data scientists work their “magic”, and then take the output at face value (without asking too many questions this time) and implement the recommendations. Now, imagine if Marketing or Sales were operating this way.
A new framework: Analytics as a business process
To address these shortcomings, we have developed our own framework, which treats analytics as a fully-fledged business process. It follows the same overall logic as the existing frameworks (which, again, is not in question) but adds several dimensions:
- First, the “Business Side” and the “Science Side” are given an equal weight in the process. It is an acknowledgement that the “Business Side” needs to be significantly more involved and truly own the analytics initiatives. In other words, we are advocating for “more business”, not “less science”.
- Second, we separate the “Framing” phase from the “Implementation” one. This separation helps acknowledge the fact that analytics is typically a “slow thinking” exercise, to borrow a well-known term from Nobel prize Daniel Kahneman. Framing the problem and its potential solution(s) should truly be at least half of the process.
- Third, we see this framework as instrumental in turning analytics as business process instead of a mere succession of independent projects. Analytics, and pricing analytics in particular, should be fully integrated into a company’s operations, not a one-time event.
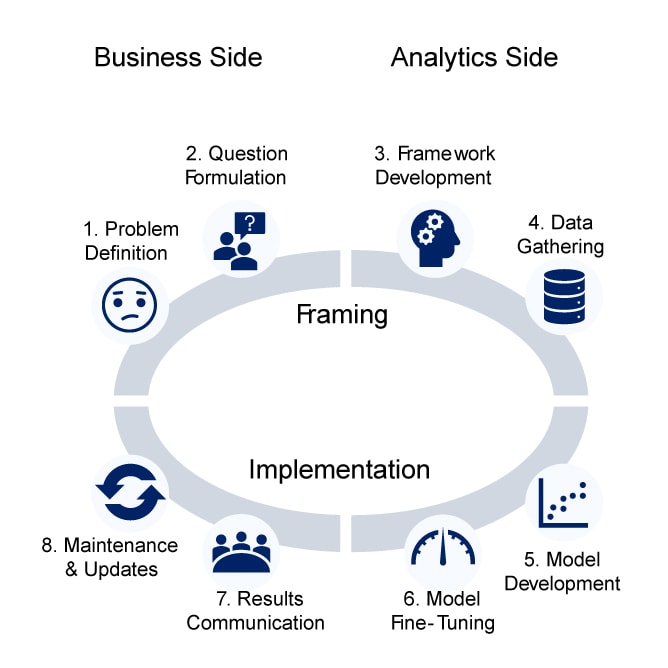
Analytics as a process
Business Framing
Like with every other business process, we start with the problem we are trying to solve. This initial stage of the Framing phase is definitely a “Business Side” activity. However, and unlike most existing analytics framework, we break this phase into two distinct steps:
1. Problem definition
This is what most practitioners think of when they see the first step in the CRISP-DM framework. It should be spelled out and written down, in plain English: what problem are you trying to solve? Proper answers could be “we are trying to grow revenue”, “our promotions are not working”, “we are losing market share”, etc.
2. Question formulation
We see this stage as different from defining the problem. “We are losing market share” is a problem. “Are our prices too high to be competitive?” is a question whose answer to may help solve the problem. There are three types of questions most frequently encountered in analytics, based on what the business stakeholders are trying to achieve:
- Learn, e.g., what are our prices relative to our competitors’?
- Understand, e.g., why are our prices higher than our competitors’?
- Decide, e.g., should we lower our prices?
3. Framework development
We see this stage as a highly conceptual and “fluid” one. This is where ideas are being shared and concepts are being built. This is also where, in an “agile” workflow, the team would come back if some of the first ideas being considered turn out to be dead ends. Two questions need to be answered at the end of this stage:
- Can the (business) question be answered by Analytics?
- If so, how are we planning to answer it?
4. Data gathering
A big part of the “how” in Analytics relies on data. Does the business even have the data necessary to answer its question? The data gathering stage involves more than the data collection itself. It also requires the data science team to conduct a thorough audit of data quality and suitability for the analysis, as well as an initial exploration of the data at hand. Expect to see a data quality report as well as some preliminary data visualizations at the end of this stage.
5. Model development
We are now at the core of a data scientist’s job: building the right model, given the question asked and the data available. It doesn’t mean business leaders should not be involved. Without getting too technical, a business leader should at least be able to determine if their data scientists are using the right class of model.
6. Model fine-tuning
Before giving a definitive answer back to the business, data scientists need to fine-tune their models. This step is iterative by nature (the first model is seldom the “right” one) and is critical to ensure that the results are robust enough to be shared beyond the data science team and eventually implemented by the business.
7. Results communication
This step is the one often leading to heated meetings, between the analytics team that tends to undersell their work and the business leaders waiting for them with sky-high expectations. Our approach is to boil it down to a simple question: did we solve the problem defined in Step 1? We also recommend distinguishing model output, results and (expected) business outcomes.
8. Maintenance and updates.
This last step might be the most difficult one, even for companies considered to be good at analytics. It usually involves a third “side”, IT, to ensure that the solution developed by the analytics team (and validated by the business) can be implemented and maintained over time and “at scale. Systems are obviously a big component of this step and the main bottleneck.