The concept of analysis emerged in Ancient Greece and literally means “the resolution of anything complex into simple elements”. The word itself is the combination of ana (“up, back or throughout”) and lysis (“to loosen, divide, cut apart”).
In other words, one analyzes a problem by decomposing it into smaller elements to understand how they are connected to each other. These elements are typically called variables or factors in modern analytics. The connection between them is what your data scientists call a model.
This definition brings home two critical ideas taught in every statistics class and (hopefully) used by all practitioners every day:
- Analytics is, fundamentally, all about understanding relations between variables. While it is always good to start with some simple, descriptive metrics (e.g. average sales, price variance, etc.), the true value of analytics lies in building models aimed at representing how these variables impact each other. The quality of these models (their accuracy) depends on how closely they represent the real-life interactions between their variables.
- The variables in a model need to be independent from each other. Going back to the original meaning of analysis helps us understand why. To truly understand (and eventually solve) a problem, one first needs to divide it into its smaller components. The division shouldn’t stop until the core elements of the problem have been identified.
These two ideas (independence and interrelations) are fundamental in analytics. However, they are not enough. The (proposed) interrelations between variables also have to make logical sense. Enters the first data scientist in history.
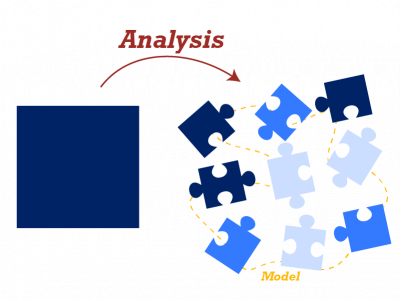
The first (known) use of the word “analytics” comes from no less than Aristotle himself, almost 2500 years ago. Interestingly, the word appeared in Aristotle’s treatise on Logic, now known as The Organon. Yes, for Aristotle, analytics belonged in logic. So much so that two of the six books in the Organon are titled “Prior Analytics” (on deductive reasoning) and “Posterior Analytics” (on demonstration and scientific knowledge).
Granted, Aristotle wasn’t building random forest models in Python but the analytical process he developed in The Organon provided the conceptual foundations for the work that modern scientists around the globe do. Critically, he emphasized the absolute necessity of using logic in any analytics endeavor.
You may be wondering: how is Aristotle’s work on Logic relevant to solving 21st-century business problems using tons and tons of data and cutting-edge technology?
Here is how: the lack of logic can lead to the wrong decisions. While this statement is certainly more applicable to politics than it is to business, companies are not immune to drawing the wrong conclusions because of a lack of logical thinking, even with (or, rather, despite) solid data and analytics to back it off. Logic is what makes the difference between simple correlations (especially the spurious ones) and truly causal relationships. Logic also takes away potential biases the business leaders could base their decision on. Finally, logic can compensate for bad or incomplete data.
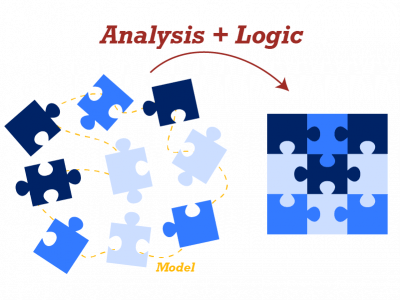